Phonological scoring of non-standard speech
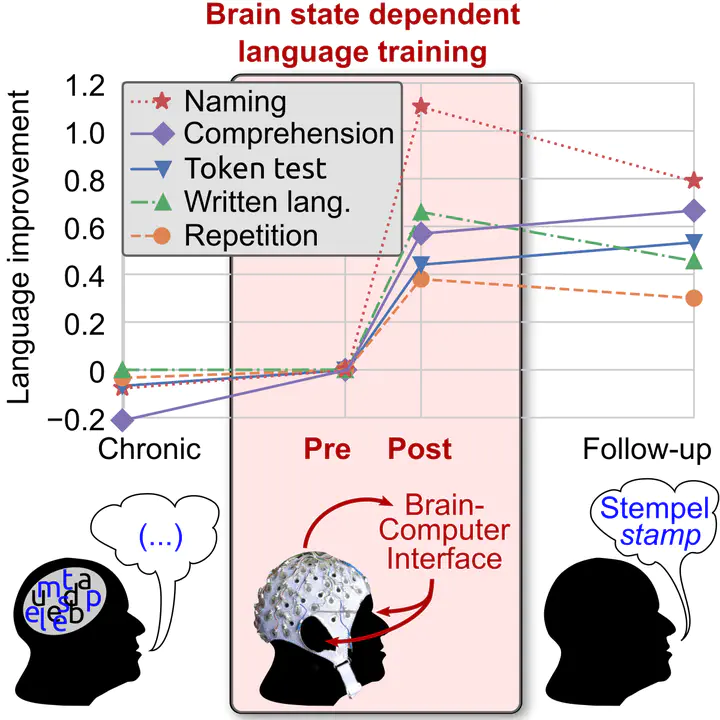
Context
While deep learning approaches have significantly pushed language applications like keyword spotting in audio recordings, natural language processing on text documents etc., these solutions for the masses can not applied directly to non-standard speech signals, e.g., audio recordings from patients with language production deficits after stroke (aphasia).
Building upon an existing keyword spotting solution for such aphasic speech recordings, the student’s task is to develop and evaluate a machine learning approach capable to deliver phonological scores of speech, which can enrich or even replace the scoring of an expert. Emphasis will be on the sample efficient use of patient’s speech data, e.g., by using pre-trained models.
Research question
How can existing pre-trained language models be modified to serve for the scoring of non-standard speech of patients with language disorders?
Skills / background required
- Very proficient in Python
- Machine learning, with rich hands-on experience in deep learning, ideally on natural speech data
- Experience in using a compute cluster