Noise-tagging
A BCI using the code-modulated visual evoked potential (c-VEP).
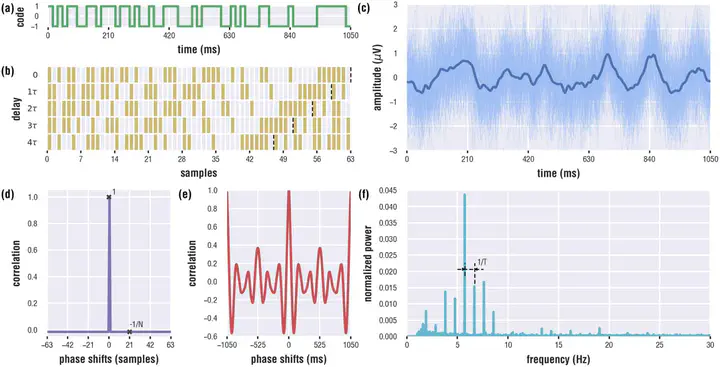
Summary
In the “noise-tagging” brain-computer interfacing (BCI) project, we utilize pseudo-random nois-codes (PRNC) as visual stimulation sequences to evoke so-called code-modulated visual evoked potentials (c-VEPs) in the electroencephalogram (EEG). These “noise-tags” exhibit a spread-spectrum signal and have godd auto- and cross-correlation properties, making them ideal as stimuli for an evoked BCI.
We have designed a generative method called ‘reconvolution’, which combines both deconvolution and convolution to learn and predict responses to these visual stimulation sequences. Specifically, adhering to the ’linear superposition hypothesis’, the complex c-VEP can be decomposed into a summation of time-shifted versions of one or several transient response(s) to individual events within the stimulus sequences (e.g., flashes).
A common application of this technique is found in the well-known matrix speller BCI for communication. A 6 x 6 grid is visually presented on a screen, with each cell containing a letter. While the user attends to a target cell, all cells start flashing concurrently with unique noise-tags. Meanwhile, the EEG is recorded from several scalp electrodes which is interpreted by the BCI. Specifically, from the EEG, the BCI decodes which noise-tag the user was attending to. By doing so, the most likely cell is selected, enabling a user to spell a certain symbol, by briefly looking at it. Afterwards, a new cycle starts, which allows a user to spell letters one by one to form words and full sentences.
Challenges
The main objective in the noise-tagging project is to create a BCI platform that decodes from EEG which noise-tag a user was attending to. The challenges are (1) accuracy, (2) speed, and (3) user-friendliness. Specifically, the BCI should be equal to or as close to 100% accuracy as possible, to prevent erroneous selections; the BCI should emit the selection as soon as possible to allow fast communication; the BCI should be plug-and-play, use a practical EEG headset with only a few electrodes, should be convenient to use, etc. Additionally, this technology allows investigating the visual system in several ways, which in turn could also lead to a better understanding of the neural mechanisms underlying visual perception. Finally, other applications are investigated such as quick brain-based diagnostics of perceptual pathways (e.g., perimetry, audiometry).
Decoding The decoding is typically achieved by applying a template-matching classifier. During training, template responses to each of the stimulation sequences are predicted by reconvolution, embedded in a canonical correlation analysis (CCA), which both learns the temporal transient responses, as well as a spatial filter to optimally make use of the multiple EEG electrodes. When a new single-trial comes in, the correlation with all templates is computed, and the argument that yields the largest correlation is selected.
Dynamic stopping When recording a new single-trial, the data are accumulated in short segments of about 100 ms. When a new segment comes in, classification is applied resulting in a selection together with a certainty value. If the certainty is sufficiently high, the trial stops and the label emitted (e.g., the letter selected), otherwise more data is collected. This method automatically emits the selection as soon as possible.
Zero-training Most BCIs require long training sessions to acquire calibration data, which are used for the classifier to learn the user-specific brain activity patterns relevant for the application. Instead, the CCA-based reconvolution allows for a straight-away plug-and-play approach without any prior knowledge (i.e., zero-training).
Stimulation parameters Typically, the noise-tags are borrowed from telecommunication (i.e., code domain multiple access, CDMA). For instance, quite typically an m-sequence or a set of Gold codes is used. These are codes that have known low auto- and cross-correlation properties. However, it remains unknown to what extent these codes remain uncorrelated in the response-domain. We are investigating alternative code sets, as well as the optimization (in real-time) of stimulus parameters, that yield maximally uncorrelated template responses. Finally, not only the stimulation sequence, but also stimulation parameters such as presentation rate, colors, textures, etc. can be optimized to improve decoding performance as well as user-comfort.
Stimulation modality Typically, the noise-tags are presented visually, which yields a gaze-dependent BCI (i.e., one that still depends on eye-movements). We are working towards a gaze-independent BCI using covert spatial attention, as well as presenting the stimuli in the auditory and tactile domain.
Key publications / awards
-
Brain--computer interfaces based on code-modulated visual evoked potentials (c-VEP): A literature review. Journal of Neural Engineering.(2021).
-
From full calibration to zero training for a code-modulated visual evoked potentials for brain--computer interface. Journal of Neural Engineering.(2021).
-
A visual brain-computer interface as communication aid for patients with amyotrophic lateral sclerosis. Clinical Neurophysiology.(2021).
- (2015).
- Our work was awarded the first price (€300k) in the ALS Assistive Technology Challenge offered by the International ALS Association and Prize4Life.
- Our work was nominated for the International BCI Award 2016.